Are you curious about RUL and how it can revolutionize your approach to decision-making, resource management, or predictive analytics? RUL, which stands for Remaining Useful Life, is a critical concept in various industries, including manufacturing, healthcare, and technology. It helps businesses and individuals predict the lifespan of equipment, tools, or systems, enabling proactive measures to prevent failures and optimize performance. In this article, we will delve deep into the concept of RUL, exploring its definition, applications, and benefits. Whether you're a professional looking to enhance operational efficiency or a curious learner eager to understand this concept, this guide will provide you with all the essential insights.
The importance of RUL cannot be overstated. It plays a pivotal role in predictive maintenance, helping organizations save costs, reduce downtime, and improve safety. By understanding how RUL works and its applications, you can make informed decisions that impact your business or personal projects. This article will provide a comprehensive overview of RUL, supported by data, expert insights, and practical examples.
In the following sections, we will break down the concept of RUL into digestible parts, covering its definition, methodologies, tools, and real-world applications. We’ll also discuss how RUL aligns with industry standards and future trends. By the end of this article, you’ll have a clear understanding of RUL and how it can be applied to your specific needs. Let’s dive in!
- Exploring The Heartwarming World Of Outdaughtered News A Deep Dive
- Unveiling The Enigma What You Need To Know About Emuyumi
Table of Contents
- What is RUL?
- Why is RUL Important?
- Methodologies for Calculating RUL
- Tools and Technologies for RUL
- Applications of RUL Across Industries
- Challenges in Implementing RUL
- Future Trends in RUL
- Case Studies of RUL Implementation
- Best Practices for RUL
- Conclusion and Call to Action
What is RUL?
RUL, or Remaining Useful Life, refers to the estimated time left before a system, component, or asset reaches the end of its operational life. This concept is widely used in predictive maintenance, where the goal is to anticipate failures and take corrective actions before they occur. RUL is calculated using various techniques, including machine learning algorithms, statistical models, and sensor data analysis.
Understanding RUL requires familiarity with its foundational principles. Here are some key points:
- RUL is a predictive metric that helps estimate when a component will fail.
- It is widely used in industries like manufacturing, aviation, and healthcare.
- RUL calculations rely on data such as usage patterns, environmental conditions, and historical performance.
How RUL Differs from Traditional Maintenance
Traditional maintenance approaches, such as reactive or preventive maintenance, often rely on fixed schedules or respond to failures after they occur. In contrast, RUL enables a proactive approach by predicting when a failure is likely to happen. This shift not only reduces downtime but also optimizes resource allocation and extends the lifespan of assets.
- Unveiling The World Of Movie Rulzcom Your Ultimate Guide To Streaming Excellence
- Unveiling The Truth Did Machine Gun Kelly Cheat
Why is RUL Important?
The importance of RUL lies in its ability to transform decision-making processes. By providing actionable insights into the lifespan of assets, RUL helps organizations avoid costly breakdowns, improve safety, and enhance operational efficiency. Let’s explore some of the key reasons why RUL is crucial:
- Cost Savings: Predictive maintenance powered by RUL reduces repair costs and minimizes the need for emergency interventions.
- Improved Safety: By predicting failures, RUL helps prevent accidents and ensures compliance with safety standards.
- Enhanced Productivity: Downtime is minimized, allowing businesses to operate at full capacity.
Impact on Industries
RUL has a profound impact on industries such as manufacturing, aviation, and energy. For example, in aviation, RUL helps airlines predict engine failures, ensuring passenger safety and reducing maintenance costs. Similarly, in manufacturing, RUL enables factories to optimize production schedules and reduce waste.
Methodologies for Calculating RUL
Calculating RUL involves a combination of data analysis, machine learning, and domain expertise. Here are some of the most common methodologies used:
- Statistical Models: Techniques like regression analysis and time-series forecasting are used to estimate RUL based on historical data.
- Machine Learning: Algorithms such as neural networks and decision trees are employed to predict RUL using real-time sensor data.
- Physics-Based Models: These models use physical principles and equations to simulate the behavior of components and estimate their lifespan.
Choosing the Right Methodology
The choice of methodology depends on factors such as the availability of data, the complexity of the system, and the desired accuracy. For instance, machine learning models are ideal for systems with large datasets, while physics-based models are better suited for systems with well-defined physical behaviors.
Tools and Technologies for RUL
Implementing RUL requires the use of specialized tools and technologies. These tools help collect, analyze, and interpret data to provide accurate predictions. Some of the most popular tools include:
- Predictive Maintenance Platforms: Software solutions like IBM Maximo and SAP Predictive Maintenance are widely used for RUL calculations.
- IoT Sensors: Internet of Things (IoT) devices collect real-time data from assets, enabling continuous monitoring and analysis.
- Data Analytics Tools: Platforms like Tableau and Power BI help visualize RUL data and generate actionable insights.
Emerging Technologies
Emerging technologies such as edge computing and blockchain are also being integrated into RUL systems. Edge computing allows data processing to occur closer to the source, reducing latency and improving accuracy. Blockchain, on the other hand, ensures data integrity and transparency in RUL calculations.
Applications of RUL Across Industries
RUL has diverse applications across various industries. Here are some examples:
- Manufacturing: Predicting machine failures to optimize production schedules.
- Healthcare: Estimating the lifespan of medical devices to ensure patient safety.
- Energy: Monitoring wind turbines and solar panels to maximize energy output.
Case Study: RUL in Aviation
In the aviation industry, RUL is used to predict engine failures and schedule maintenance activities. For instance, airlines use RUL to monitor jet engines, ensuring they operate efficiently and safely. This not only reduces maintenance costs but also enhances passenger safety.
Challenges in Implementing RUL
While RUL offers numerous benefits, its implementation is not without challenges. Some of the common obstacles include:
- Data Quality: Inaccurate or incomplete data can lead to unreliable RUL predictions.
- Complexity: Developing and deploying RUL models requires expertise in data science and engineering.
- Cost: Implementing RUL systems can be expensive, especially for small and medium-sized businesses.
Overcoming Challenges
To overcome these challenges, organizations can invest in training programs, adopt scalable solutions, and collaborate with industry experts. Additionally, leveraging open-source tools and cloud-based platforms can reduce costs and improve accessibility.
Future Trends in RUL
The future of RUL is promising, with advancements in technology driving innovation. Some of the key trends include:
- AI and Machine Learning: Enhanced algorithms will improve the accuracy and reliability of RUL predictions.
- Digital Twins: Virtual replicas of physical assets will enable real-time monitoring and simulation.
- Sustainability: RUL will play a crucial role in achieving sustainability goals by optimizing resource usage.
Impact on Society
As RUL becomes more widespread, its impact on society will grow. From reducing carbon emissions to improving healthcare outcomes, RUL has the potential to transform industries and improve quality of life.
Case Studies of RUL Implementation
Let’s explore some real-world examples of RUL implementation:
- Case Study 1: A manufacturing company used RUL to predict machine failures, reducing downtime by 30%.
- Case Study 2: A healthcare provider implemented RUL to monitor medical devices, improving patient safety and compliance.
- Case Study 3: An energy company leveraged RUL to optimize wind turbine performance, increasing energy output by 20%.
Best Practices for RUL
To maximize the benefits of RUL, organizations should follow these best practices:
- Data Collection: Ensure high-quality data is collected consistently.
- Model Validation: Regularly validate and update RUL models to maintain accuracy.
- Collaboration: Foster collaboration between data scientists, engineers, and domain experts.
Conclusion and Call to Action
RUL is a powerful concept that can transform industries by enabling predictive maintenance, optimizing resource usage, and improving safety. By understanding its methodologies, tools, and applications, you can harness the full potential of RUL to achieve your goals. Whether you're a business owner, engineer, or data scientist, RUL offers valuable insights that can drive success.
We encourage you to share your thoughts and experiences with RUL in the comments section below. If you found this article helpful, don’t forget to share it with your network. For more informative content, explore our other articles on predictive analytics and data-driven decision-making.
- Noah Wyles Voice Change The Surprising Evolution Of An Iconic Sound
- Jeff Dunhams Wealth Empire Unveiling His Net Worth In 2024
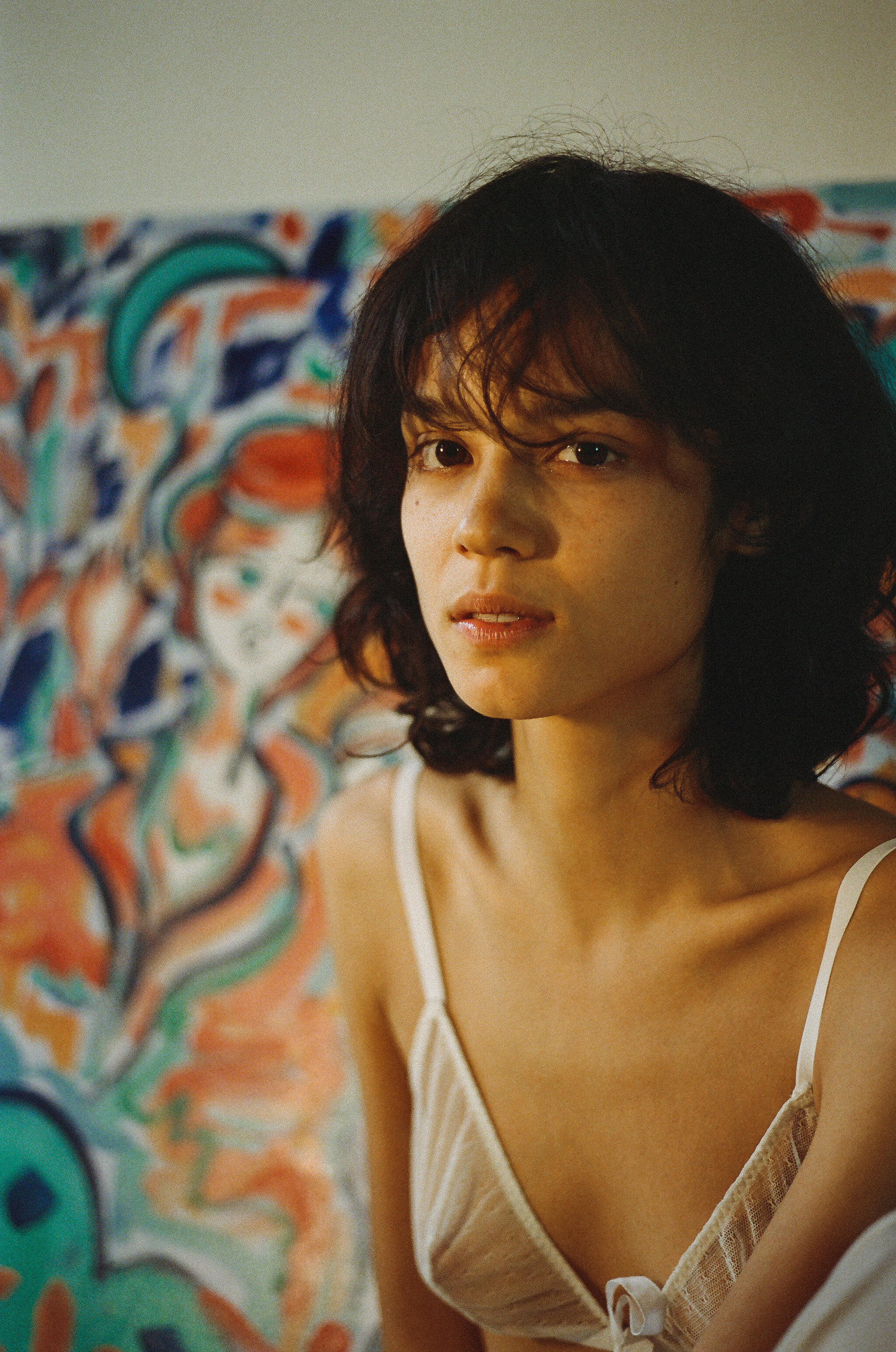.jpg?format=1500w)
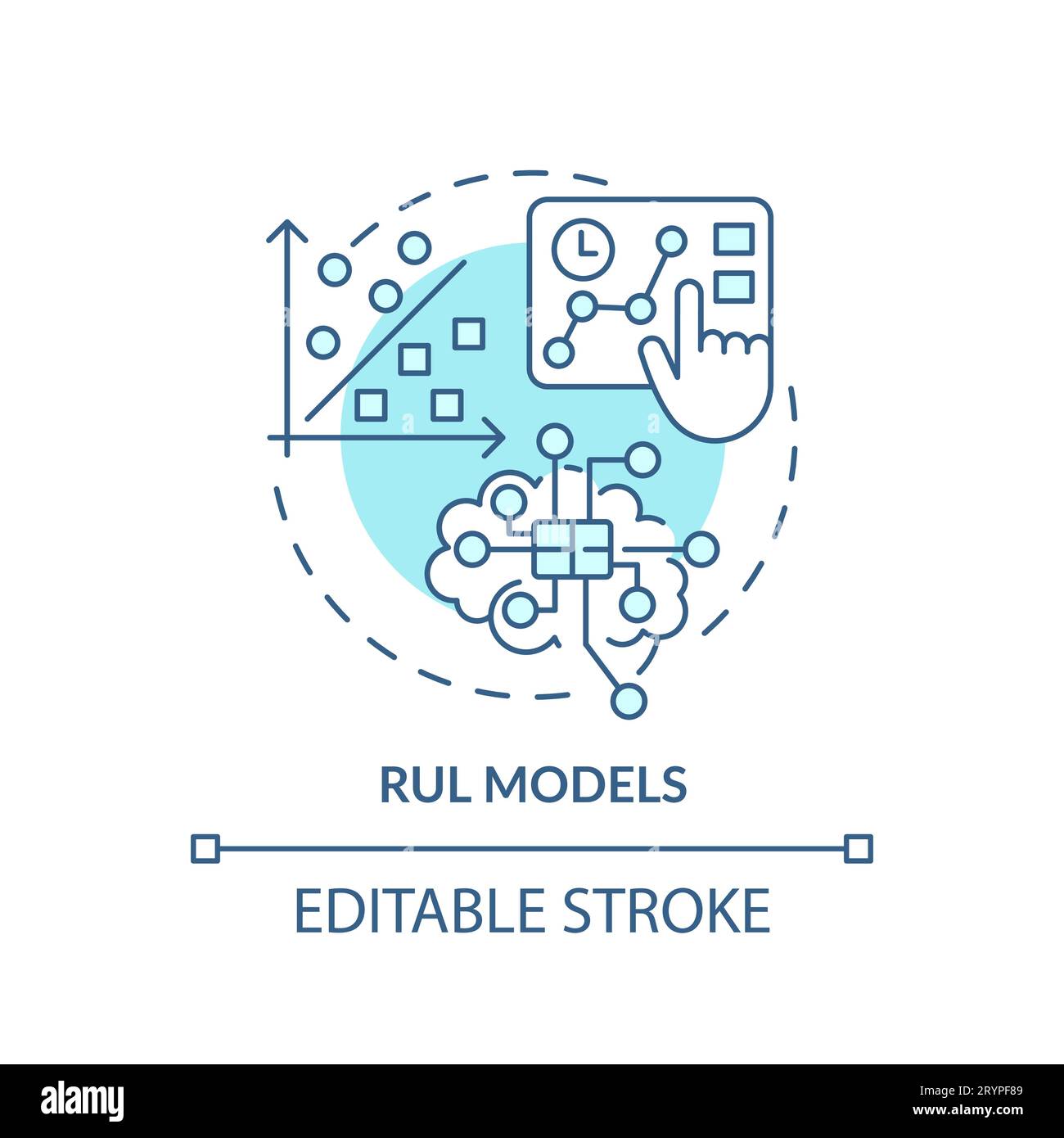